KS Park’s comment at Korea AI Summit on December 15, 2022.
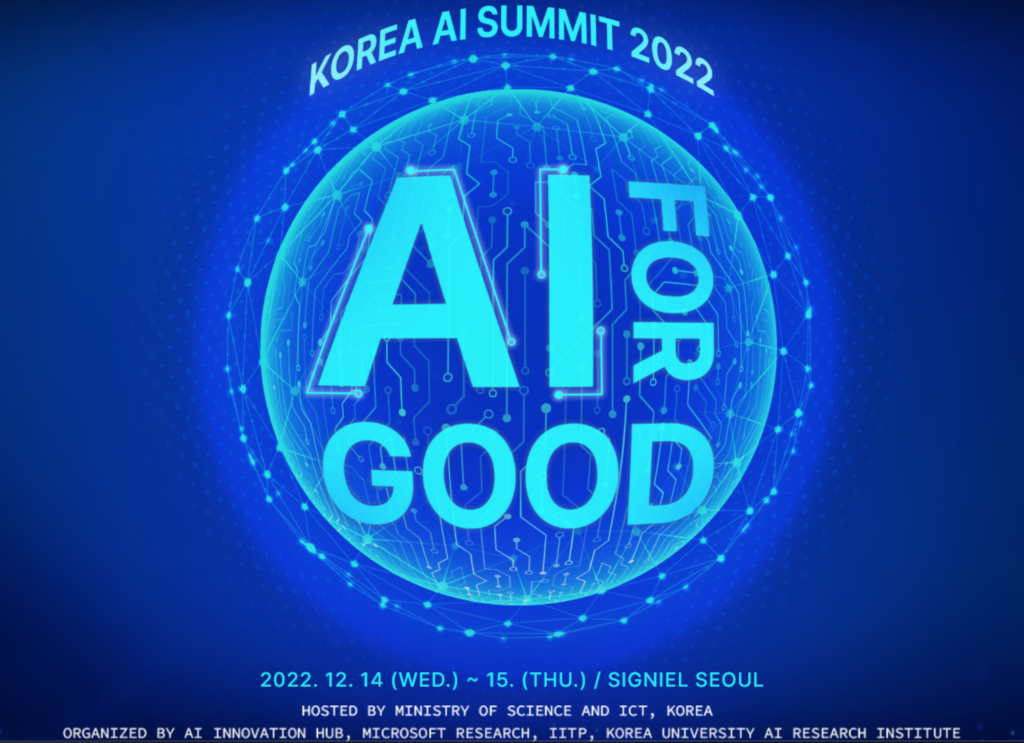
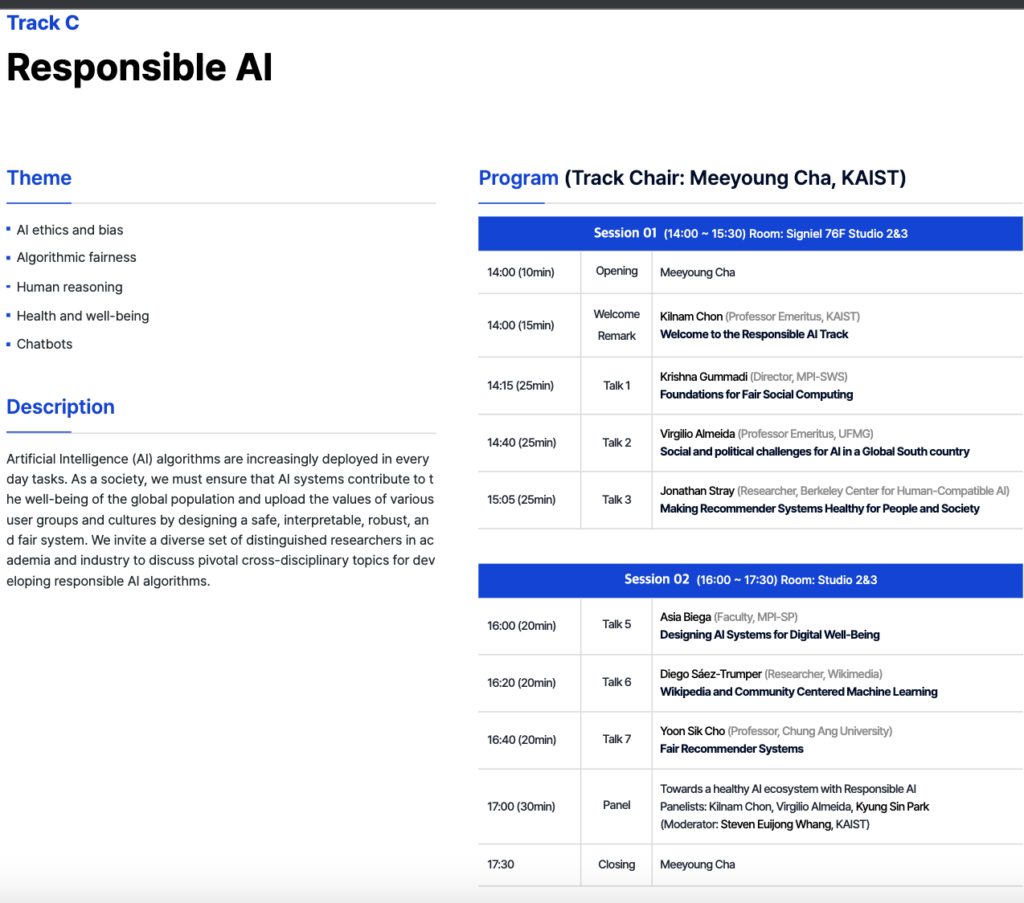
Computers scientists have become philosophers and lawyers. They are trying to define mathematically what fairness means, what discrimination means, etc., so as to make artificial intelligence fair. I think that there is a room for much collaboration in this area. I have the fortune of being a physics major and I used that quantitative learning in the field of law trying to develop mathematical formula defining constitutional violations.
Yet my conclusion is that there is no scientifically derivable concept of discrimination. Is discrimination , is it necessarily bad? What do we mean by having a “discriminating taste”? We want the right to be discriminating in marital choices, and other most basic human choices. Actually, the core of our conceptions of liberty includes the right to discriminate in certain areas of life. There are substantive moral decisions that need be made to decide what discrimination means, which cannot be calculated by pure logic but be made only by humans.
Therefore, many of the issues discussed earlier such as how and whether to make AI more responsible are not AI issues but human issues. Current version of AI is machine learning. whatever human bias and frailties will go into training data. Microsoft Chatbot Tay and Iruda are prime examples. Yet we do not stop learning from ourselves. Look at MIT’s Moral Machines. The idea is to use the data collected there for self-driving machines. We want machines to replicate our behavior. If we want AI to be good, we ourselves should define what that good is. Also, as long as AI remains a machine learning from the big data of human behavior, we ourselves should behave better.
One low-hanging fruit from this discussion is to make the training data more diverse. Facial recognition AI is notoriously failing in recognizing racial minorities’ faces, Amazon’s hiring software was pulled off after having shown discriminatory tendencies against female applicants, and finally Chatbot Tay also immediately showed racist and sexist tendencies. The underlying causes of these three failures are closely related to the insufficiency of balanced and diverse data about racial minorities, successful career women, and anti-discriminatory tweets, respectively. To make AI fairer in the above instances, we need to collect more data about and from racial minorities, successful career women, and anti-discriminatory Twitterers.
The most significant obstacle to such data diversification is data protection laws. Data minimization is one of the principles of protection of personal data. However, we should break down data minimization into vertical minimization and horizontal one. Data minimization aims to reduce the risk to privacy when data collected deeply into each individual. It does not concern collection of data about large number of people if data collected from each individual are at a shallow level. For instance, if data collected are in anonymized or pseudonymized form, collecting data from a large number of people will not necessarily contradict the data minimization principle. GDPR was innovative in this regard by concocting the concept of pseudonymized data which GDPR allowed to be processed for scientific research without data subjects’ concent . Korea adopted that innovation and received EU’s adequacy decision early this year.
Another obstacle is data monopoly whereby a small number of platforms for online human behavior control vast amounts of personal data. Data portability, another innovation of GDPR, allows data subjects to transfer data from platforms to platforms and also entrust with AI researchers. AI researchers can diversify their training data for their AI software by purchasing the data from data subjects.
Data governance is a key to creation of responsible and fair AI. Distinguishing horizontal data minimization from the vertical and enhancing and data portability will be important enablers in creating rich and diversified training data, a prerequisite for a responsible and fair AI.
0 Comments